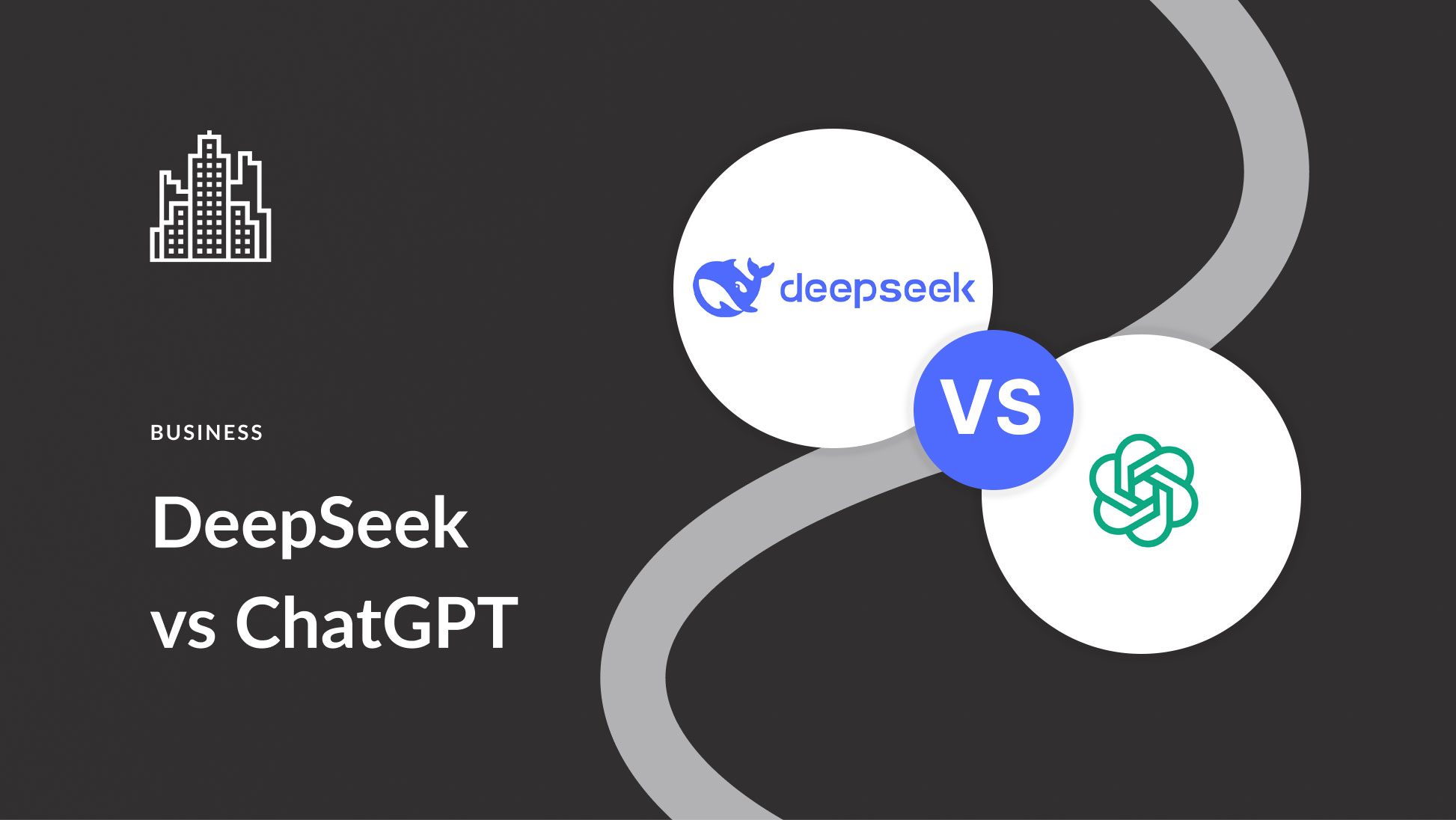
DeepSeek’s AIs: What humans really want
DeepSeek Cracks a Longstanding AI Challenge with Breakthrough in Reward Modeling
Chinese AI startup DeepSeek, in collaboration with Tsinghua University, has unveiled a major breakthrough in AI reward modeling—an area that has long stumped researchers. This advance could significantly improve how AI systems reason, learn from feedback, and generate more human-aligned responses.
Their new technique, detailed in the paper “Inference-Time Scaling for Generalist Reward Modeling”, claims to outperform existing methods and delivers “competitive performance” compared to leading public reward models.
At its core, the innovation enhances how AI interprets and aligns with human preferences, a critical element in building more trustworthy and capable AI.
What Are Reward Models, and Why Do They Matter?
In reinforcement learning for large language models (LLMs), reward models act like digital instructors—offering feedback signals that guide the model toward desirable behavior. These models are essential for teaching AIs to respond in ways that reflect human intent, especially as applications extend beyond simple Q&A into complex reasoning and decision-making.
As DeepSeek’s researchers explain, “reward modeling is a process that guides an LLM towards human preferences.” But existing models often falter when navigating open-ended, ambiguous, or nuanced queries across diverse domains.
A Dual Approach to Smarter Rewards
DeepSeek’s breakthrough relies on two complementary methods:
-
Generative Reward Modeling (GRM): Unlike traditional scalar methods, GRM uses language to express richer, more nuanced reward signals. It’s flexible and allows dynamic scaling at inference time—meaning models can adapt performance based on available computing power.
-
Self-Principled Critique Tuning (SPCT): This reinforcement learning strategy trains the model to generate its own principles and apply them when evaluating AI responses. It creates a loop where the model adapts and refines its reward judgments based on context.
Zijun Liu, co-author of the paper and researcher at DeepSeek and Tsinghua University, explains that this approach allows reward principles to be generated “based on the input query and responses, adaptively aligning the reward generation process.”
One of the most notable aspects of the method is “inference-time scaling”—improving model performance by allocating more compute during response generation, not just during training. The research shows that even with smaller models, scaling at inference time can outperform larger models trained with conventional methods.
Why This Matters for the AI Industry
DeepSeek’s innovation arrives at a critical point in AI’s evolution. As reinforcement learning becomes the backbone of post-training for LLMs, reward modeling is more important than ever in aligning models with human values, enabling complex reasoning, and adapting to diverse environments.
Potential implications include:
-
More accurate feedback: Improved reward modeling results in more precise training signals, leading to better AI behavior.
-
Greater adaptability: Inference-time scaling gives models the flexibility to perform well even with limited training size.
-
Wider applicability: AI systems become more capable in complex, less-structured domains.
-
More efficient AI development: Smaller models can match or exceed larger models’ performance when paired with smart inference-time strategies—lowering cost and compute needs.
DeepSeek’s Momentum in the AI Race
Founded in 2023 by entrepreneur Liang Wenfeng, Hangzhou-based DeepSeek is quickly gaining global attention. Its recent DeepSeek-V3-0324 upgrade introduced improved reasoning, better front-end development capabilities, and enhanced Chinese language proficiency.
The company is also doubling down on its commitment to open-source AI—releasing five codebases in February and promising future access to the GRM models, though no specific timeline has been shared yet.
Speculation is also mounting over the release of DeepSeek-R2, a successor to the current R1 reasoning model. While Reuters has reported on possible timelines, DeepSeek has yet to make an official statement.
The Future of AI Reward Models
DeepSeek’s work reinforces a growing realization: progress in how AI learns can be just as impactful as building bigger models. By improving the quality of feedback and making learning more scalable, researchers are helping to create AI systems that are better at understanding—and aligning with—what people actually want.
As reward modeling continues to evolve, innovations like DeepSeek’s could redefine how we train, deploy, and trust AI in the years ahead.
1 Comments
Moolokai
April 14, 2025Nice!